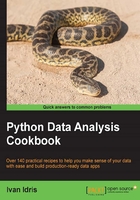
Data analysis, data science, big data – what is the big deal?
You probably have seen Venn diagrams depicting data science as the intersection of mathematics/statistics, computer science, and domain expertise. Data analysis is timeless and was there before data science and even before computer science. You could do data analysis with a pen and paper and, in more modern times, with a pocket calculator.
Data analysis has many aspects, with goals such as making decisions or coming up with new hypotheses and questions. The hype, status, and financial rewards surrounding data science and big data remind me of the time when datawarehousing and business intelligence were the buzz words. The ultimate goal of business intelligence and datawarehousing was to build dashboards for management. This involved a lot of politics and organizational aspects, but on the technical side, it was mostly about databases. Data science, on the other hand, is not database-centric and leans heavily on machine learning. Machine learning techniques have become necessary because of the bigger volumes of data. The data growth is caused by the growth of the world population and the rise of new technologies, such as social media and mobile devices. The data growth is, in fact, probably the only trend that we can be sure of continuing. The difference between constructing dashboards and applying machine learning is analogous to the way search engines evolved.
Search engines (if you can call them that) were initially nothing more than well-organized collections of links created manually. Eventually, the automated approach won. Since, in time, more data will be created (and not destroyed), we can expect an increase in automated data analysis.